In today’s post-COVID world, the retail landscape in terms of brick and mortar investments has changed forever. Some household name brand retailers have shut their doors completely and re-emerged in a different form. (See Impacts of the Covid Pandemic on Retail Brick and Mortar blog post). The latest example is Bed, Bath & Beyond in Canada. They declared bankruptcy and shuttered all brick and mortar stores early this year. However, opportunity knocks as Overstock.com, an online furniture retailer in the US has now purchased all digital assets and will rebrand Bed, Bath & Beyond as an online only play. Given the growth in the home retail category over the 2019-2022 period, this was a bit of a surprise. Yet in other stories, some retailers have emerged stronger and are expanding brick and mortar plays through acquisition (Rogers & Shaw, Empire - Longo’s and Farm Boy, etc.), new build expansion (Ren’s Pets and Firehouse Subs), retail partnerships (Staples and Bell; Walmart and Sleep Country) and investments in store renovations through digital technology (Canadian Tire, Metro, Loblaws) to refresh their brands and optimize their return on investment. But none of this is new of course - some of it was predictable while others not so much.
Clearly, this is just the evolution of business strategy. Those that have adapted are building a real estate strategy process that is flexible, sustainable, reliable and sensitive to ever-changing retail and consumer trends over time. But how do you stay nimble, future looking and data-driven at the same time? A lot is talked about being “data-driven”. The nature of data–driven is that it is historical - backward looking. Important yes, but sometimes the data misleads (e.g. the pandemic was not predicted and it had a profound effect on retail as we knew it). So how do we account for future potential shifts in the market to compliment a data-driven retail strategy function?
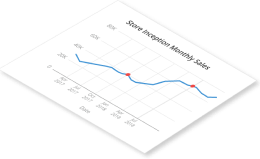
The emergence of GeoAI
GeoAI is an emerging area of discussion. Technically, it is the integration of two well known capabilities - Geospatial Data Analysis (or GIS) and Artificial Intelligence technologies. GeoAI put simply is a tool in an AI modelling and analysis system that integrates geospatial data such as mapping coordinates for stores and road systems and integrates that with other data like mobile foot traffic, historical store sales, store details like “box” square feet and department size, the penetration and growth of online delivery, etc. to build predictions about new situations, such as the potential for a new store or a renovation and their impact on the overall profitability of the store network. (See Esri article).
The challenge is that most retail real estate organizations are still using traditional GIS applications and MS Excel tools to run demographic reports to do site selection. Few have taken it to the next level which is to have the ability to do future scenario prediction. For that, GIS and Data Science teams need to work together more directly or invest in technologies that offer a GeoAI capability and can enable network scenario planning to inform strategy.
Network planning is complex and simulating net-effects is key
Scenario planning in business has been around for a long time. The tools used to produce predictions about a particular real estate scenario are evolving as GeoAI matures. Retail real estate executives are required and measured on their ability to deploy capital to the brick and mortar network with profitable outcomes. As such, having a capability to “simulate” potential scenarios is emerging as a necessary capability.
Simulating scenario outcomes is part science and part art. The technical engine should get you most of the way there based on historical information, but then having the ability to adjust those predictions for different economic scenarios (e.g. recession probability, inflation, consumer spending declines, etc.) is where the “art” or business acumen comes in. Scenario simulations allow users to chain together any series of real estate changes that can happen in a store network and measure the net-effect on a selected performance metric.
- Simulate Store Openings: You want to predict net-sales impact by measuring incremental, mature store sales combined with potential cannibalization to your existing network.
- Simulate Store Closures: Retailers are choose to close underperforming stores all the time, the question is can you understand the net effect on your network - can you recapture lost customers to nearby locations? Make sure to understand your sales transfer due to a store closure and where those sales will go: your sister stores or your competition?
- Simulate Store Relocations: Relocations can be simulated by measuring the “net sales/profit” impact captured by an opening and a simultaneous closing?
- Simulate Store Renovations: These are the most difficult predictions to make. Further, do you have enough historical data on “renovations” to make a calculation on the net-sales improvement? Even if you do, not all renovations are alike. This is where the “art” comes in! The simulations based on business rule calculations can be added to the machine learning algorithm to provide decision makers ultimate flexibility when running scenarios.
- Simulate Store Consolidations: The market has changed; and the market no longer supports two or more stores. Consolidation decisions can be simulated by estimating the “sales transfer or recapture” from closing a store by the one(s) still open and nearby.
- Banner Realignment: Larger enterprise retailers and QSR’s are multi-banner organizations. Knowing where each brand in your portfolio should be located whilst not cannibalizing the others is tricky. The simulations capability must be able to predict where the “value brand” should be and where the “premium brand” should be based on the net sales lift predicted from the models.
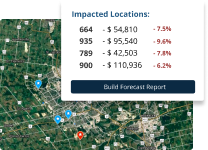
Chances are you’ve encountered all of these decisions at one time or another. It’s likely your internal real estate teams, working together with the finance department, come up with calculations for a business case. These days, the game is getting more sophisticated, and the use of the GeoAI tools is fast becoming best practice. Having a simulation capability based on the embedded ML models, helps sort through the complexity of the interactions and can give you insights and direction.
Network Simulations: The Tools that Make it Happen
The term optimization suggests moving toward the one stable outcome from which you can’t improve any further, ”all else held equal”. This is a scientific definition but is difficult to apply in business decision-making, as we know that world events, consumer behaviour, and competitor actions are continually evolving. That is, it’s a moving target!
As such, we think of store network optimization as a process by which multiple changes to an existing network of stores have a predicted net positive impact on organizational profitability with a margin of error, subject to market constraints, such as changing consumer behaviour, market saturation, store unit performance, and predictable competitor responses and impacts. Hence, the process of “optimizing” is driven by a strategy through executing manageable ongoing adjustments toward higher performance and an ultimate goal.
This is where Network Simulations comes in - a customized version of GeoAI for your organization. It’s a new combination of data, machine learning methods, and interactive scenario planning that can inform retailers seeking optimized performance across an evolving omnichannel network. The tools in this endeavour are built using modern machine learning algorithms and include, but are not limited to:
- Sales prediction models,
- Cannibalization (sales transfer) models,
- Store Closure Models (sales transfer and cannibalization),
- Department level shelf space models.
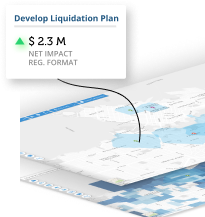
Each of these have a specific purpose in the simulation algorithm. The model outputs are the inputs to the simulation algorithm in the GeoAI world. All of these models are “trained” on the back of an integrated geospatial and proprietary organizational data sets based on historical store and network change events and outcomes.
- Sales forecasting machine learning models have been used for decades, and in retail, are a well-understood field of practice is evaluating new store success and replicating it in a geospatial platform. The main purpose of these models is to predict the potential sales at a new location from day zero to maturity, at maturity, and from maturity onwards.
- Cannibalization (sales transfer) In mature networks looking for infill, or other changes like replacing or closing poor performers, the sales forecasting capability is not enough - enter the cannibalization model. These Machine Learning models are built on historical patterns of sales changes to existing stores when a new store opens up in the same trade area.
- Closure Models are simply the estimation of the sales transfer to other stores as a result of shuttering a store location within the network, or the cannibalization of customers by competitors that are closer.
- Department level shelf space models are models that can predict the optimal shelf space by department. For example, in grocery, adding an extra 100 linear feet of space for the produce department within a fixed store footprint has a negative impact on other surrounding departments that have to shrink as a result. Finding the “best fit” linear feet for each department is a process optimizing those choices to maximize revenue and profit.
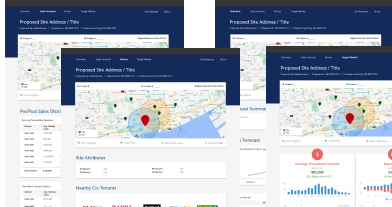
These machine learning models work together to establish the “net sales/profit impact” of a real estate event or sequence of events In mature, larger networks, overpopulating territories is a real threat to store value and the organization as a whole. All four machine learning models (and others depending on sector) are used in the simulation engine to provide insight into the net revenue or profit impacts of real estate decisions and events.
Conclusion
We all know that the formation of a strategy, especially for real estate networks, requires choices to be made. The execution of the strategy is expensive so what if you had the capability to “simulate scenarios” that are aligned with your strategic choices? The new wave of GeoAI methods are here to stay and Network Simulations are the new way to execute and adjudicate strategic choices. As a retail real estate executive, this gives you the power of scenario planning at your fingertips and facilitates the consistent communication of data-driven insights and your recommendations to truly shine in the boardroom. Using network simulations, retailers can make better-informed decisions about their real estate strategy, avoid costly mistakes, and optimize their physical spaces.
At PiinPoint, we have been using machine learning and geospatial analytics (GeoAI as it is now called) since our inception in 2014. Our Network Simulations application reduces the complexity in the market by combining trusted insights with an easy-to-use tool.
Learn how Network Simulations will improve your expansion efforts. Get a Demo today!
Photo by GeoJango Maps on Unsplash